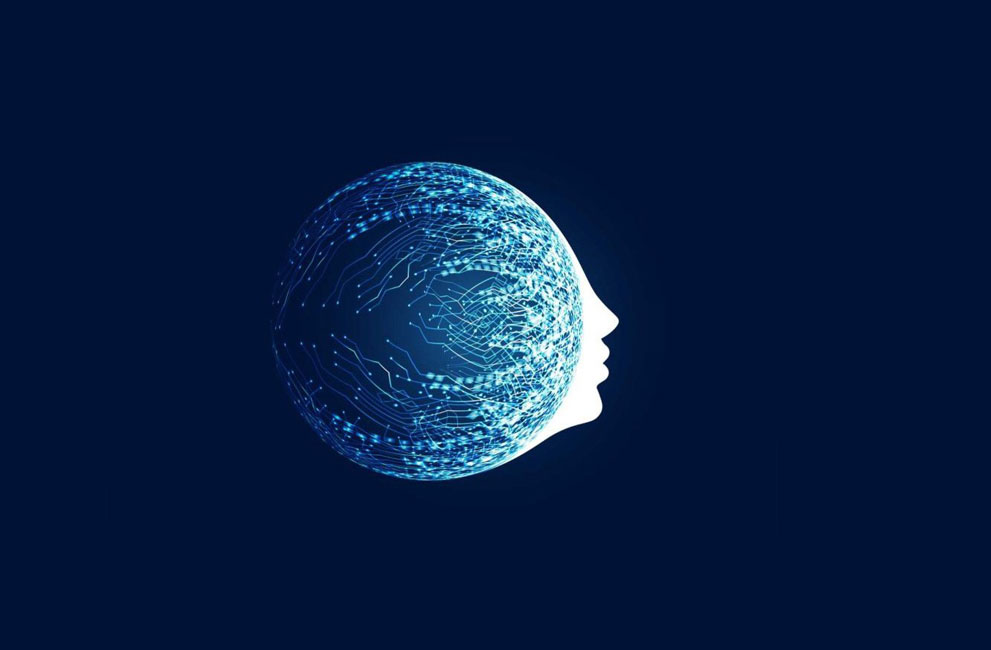
MANAGING DATA ECOSYSTEM
In 2001, William S. Cleveland used the term “Data Science” in a publication titled “Data Science: An Action Plan for Expanding the Technical Areas of the Field of Statistics”. 2 decades later the data science and related homonyms have become ubiqutous. It is interesting to give it a thought how we have comes this far in just 2 decades and data has become so powerful that quotes like “Data is the New Oil” have become a cliche.
REASONS FOR SURGE OF DATA
The reasons for surge of data science, data analytics data..whatever can be broken down in three parts: Sources, Tools, Influences.
- Sources of Data
- Internet: Phenomenal increase in use of internet both by corporates as well as consumers have created infinite and mostly unorganised data
- IoT & Mobile: Usage of Mobile and conncted devices and shifts towards mobile based platforms have further increased the generation & accumulation of transaction based, consumer profile based & machine based data
- Social Media: The explosive surve of adoption of social media across the value chain of society is generating data exponentially
- Internal: Organizations are better equipped with facilitative hardwares and softwares to store, preserve and as well as supplement their own proprietary data with external data.
- Tools
- Open Source: The rise of platform and unanimous acceptance of technologies like, R & Python well reinforced by thousands of rick packages developed by millions of briliant developers and scientists have increased the power of Open Source computing like never before and it is rising every day.
- Bundled offerings & upgrades: Propreitary software and servicve providers are developing and offering peripheral products and services to consumers for data managpement/analysis.
- Economics: The effective and accesible open source technoogies as well as mature cloyg based platforms have made the economics pretty attractive given the befits a robust data ecosystem can offer.
- Influences
- Success Stories & Marketing: Needless to say there is no dearth of success stories and white papers as well as number of startups coming up every day which are related to data. Social proof is a big influencer. Data Science or Data whatever, does sound cool.
5 KEY AREAS WHERE DATA SCIENCE IS MOST EFFECTIVELY LEVERAGED
- Decision Support in the form of BI – for making informed decisions
- Assesing Business decisions
- Sharpening up proposition of products or services
- Predict parameters of interest
- Cut Costs by Automation
IMPORTANCE OF HAVING ECOSYSTEM APPROACH TOWARDS GETTING DATA READY
Quite often organizations especially in developing economies and sectors which are typically laggards in terms of technology infusion, take up data projects in a fragmented fashion. These projects and applications are usually aimed towards solving most burning and most obvious use case. Over time a cobweb of a number of applications like, RPA tools , Image recognition based applications, customer service chatbots etc get populated. One of the very early problems with this approach is the the divergent and asynchronized nature of these applications demand to create dedicated systems and processes to serve them in terms of data & infrastructure. One of the major reason for this is presence the multiple systems across departments and multiple data sources often not in sync with each other.
It is so ironical that in many cases Data driven & Automation projects become most manual! It is like sitting in a car and hiring people to push the car forward as you don’t have the fuel.
Having a complete Digital Strategy designed with an ecosystem view for Data is the only way to become data ready and data capable. For Data Science projects to meaningfully add economic value it is imperative to think of it in terms of complete business transformation & change management rather than fragmented applications and use cases.
One of the most cross-functional & multi-disciplinary field is data science, yet it is often misinterpreted by assuming that experts from these fields just live in R, Python and Excel. If you ask even a naive practitioner of data analysis, most of her/his time is deployed in just ensuring good quality of data. If looked at it from this perspective, it is the obvious need to have a complete Data ecosystem Architecture in place with layers of Data Sources (ERPs), Databases, Data Warehouses and various applications all integrated seamlessly. The most important word here is “integrated”, you miss this and the strategy goes for a toss. The choice of technology for each layer must be seen in the context of the business & industry. The core Data Strategy must clearly define the goals, capabilities and the choice of various platforms for Analyics, BI, ML, etc.
HIGH LEVEL GOALS & USE-CASES
With Data strategy and a blueprint of Data Ecosystem in place, a high level view of what kind of problems organization would like to address using data projects and what are the potential use cases. An industry benhmarking with developed economies and sector leaders can be a good start. Some of the most prevalent use cases are:
- Predictive Analytics
- Forecasting
- Consumer Behaviour study
- Customer Service
- RPA deployment to operations
- Fraud Detection
- Social Media Marketing
- STP Analysis
- Product Recommendation
- Process Optimization
- Product Development
- Sentiment Analysis
- Natural Language Processing
- Price Optimization